- Home
- Resource Center
- Articles & Videos
- Managing AI in Localization: Principles of Prompt Design
26 May 2025
Managing AI in Localization: Principles of Prompt Design
The integration of artificial intelligence into localization workflows marks a significant advancement in linguistic technology. As large language models (LLMs) and multimodal AI systems become increasingly sophisticated, the precision and structure of prompt design have emerged as critical factors in optimizing model performance.
Watch the presentation "How to Tame your AI – A Crash Course in Prompt Design" given by Balázs Kis (memoQ) and Marina Pantcheva (RWS) at GALA 2024 Valencia. Alternatively you can read the summary underneath.
Mono-Modal vs. Multi-Modal AI
AI models can generally be categorized into mono-modal and multi-modal systems.
Mono-Modal AI and its role in localization
Mono-modal AI refers to generative models that operate with only one type of input. These models process text prompts and produce text-based responses. They cannot interpret or generate images, audio, or other data types. They strictly work within the boundaries of written language.
While powerful, mono-modal AI comes with certain limitations. Because it relies solely on text, it can struggle with context-dependent meanings, where the same word or phrase might have different interpretations based on cultural or linguistic factors.
For instance, a model trained on text alone may misinterpret a phrase if it lacks surrounding context. Additionally, mono-modal AI cannot verify non-textual details, which may be important in localization tasks such as subtitling, marketing adaptation, or cross-cultural content creation.
Multi-Modal AI and its role in localization
Multi-modal AI is designed to handle multiple forms of information. These models can take in text, images, or audio and generate outputs that span different formats.
For example, some AI systems allow users to input text and receive an image in return, while others can process an image and generate a textual description.
AI models that handle audio can convert spoken words into written text. This makes them particularly useful for speech-to-text transcription.
In localization, multi-modal AI opens up new opportunities. For instance:
- Image-to-Text Processing: AI can analyze an image and describe it in different languages. This makes it useful for accessibility and content adaptation.
- Speech Recognition and Transcription: AI can convert spoken dialogue into text, assisting with subtitle creation and multilingual voice-over workflows.
- Cross-Modal Translation: Some models allow users to input audio and receive a translated text output, addressing language gaps in real-time conversations.
Multi-modal AI is a step toward making AI more intuitive and adaptable across different use cases. However, while these systems are more flexible than mono-modal AI, they still require careful prompt design to produce accurate, contextually appropriate outputs.
Core principles of effective prompt design for localization
Before crafting prompts, it's essential to understand the different reasoning patterns AI models use to generate responses. The way a prompt is structured directly affects the quality of the output.
Some key principles of prompt design include:
- Providing clear instructions: The more specific the prompt, the better the AI’s response. For example, instead of asking an AI to “translate this sentence,” it’s more effective to specify the desired language, tone, and context.
- Using step-by-step reasoning: AI often performs better when guided through a logical sequence. For example, breaking down a complex localization task into smaller steps can improve the accuracy of the AI-generated content.
- Defining constraints: Setting boundaries for what AI should or shouldn't include in its response helps refine outputs, ensuring translations align with cultural and linguistic expectations.
By applying these principles, localization professionals can enhance the quality of AI-generated translations, transcriptions, and adaptations, ensuring content remains accurate and culturally relevant.
Types of prompts

Prompts can be categorized based on different aspects, including process, task type, format, and variance. Let’s focus primarily on format and variance, as these are crucial for designing effective AI prompts.
Format-based prompt categories:
Close-ended prompts
These prompts require the AI to choose a response from a predefined set of options.
Example: If an AI is evaluating a translation, the user may ask it to return only “true” (if the translation has an error) or “false” (if it doesn’t).
The challenge is ensuring that the AI returns responses in the correct format rather than answering with variations like “Yes” or “No.”
Benefit:
- A well-structured prompt minimizes such inconsistencies.
Open-ended prompts
These allow the AI to generate responses freely, without being limited to a fixed set of answers.
Example: “Analyze the quality of this translation and report any issues.”
Benefit:
- The AI can provide a wide range of answers, making it more useful for creative or analytical tasks.
Variance-based prompt categories:
Master prompts
These are general-purpose, reusable prompts designed to work across various languages or datasets.
Example: A master prompt for translation evaluation might be written once and applied to any language pair without changes.
Benefits:
- Scalability: The prompt can be used across various languages or tasks.
- Consistency: This prompt ensures uniform results without the need to change prompts frequently.
- Challenge: Some languages have unique characteristics that might require adjustments, meaning a master prompt may not always be effective.
Customized prompts
These are tailored prompts, created when the master prompt does not function well for a specific language or dataset.
Some languages have unique grammatical structures, idiomatic expressions, or translation challenges that require customized instructions to improve AI performance.
While Customization increases accuracy, it also reduces scalability and adds complexity to prompt management.
The future of AI in localization and the role of prompt engineering
AI is rapidly evolving, and its role in localization is expanding. While mono-modal AI has been useful for text-based tasks, multi-modal AI is set to redefine how localization professionals work with different types of content.
As AI capabilities grow, localization experts will need to adapt by:
- Refining prompt design techniques to get the most accurate and culturally appropriate AI outputs.
- Understanding AI limitations and incorporating human oversight to address errors.
- Leveraging multi-modal AI for tasks beyond translation, such as image localization, automatic subtitling, and speech recognition.
Expand Your Localization Expertise. Subscribe to Our Newsletter!
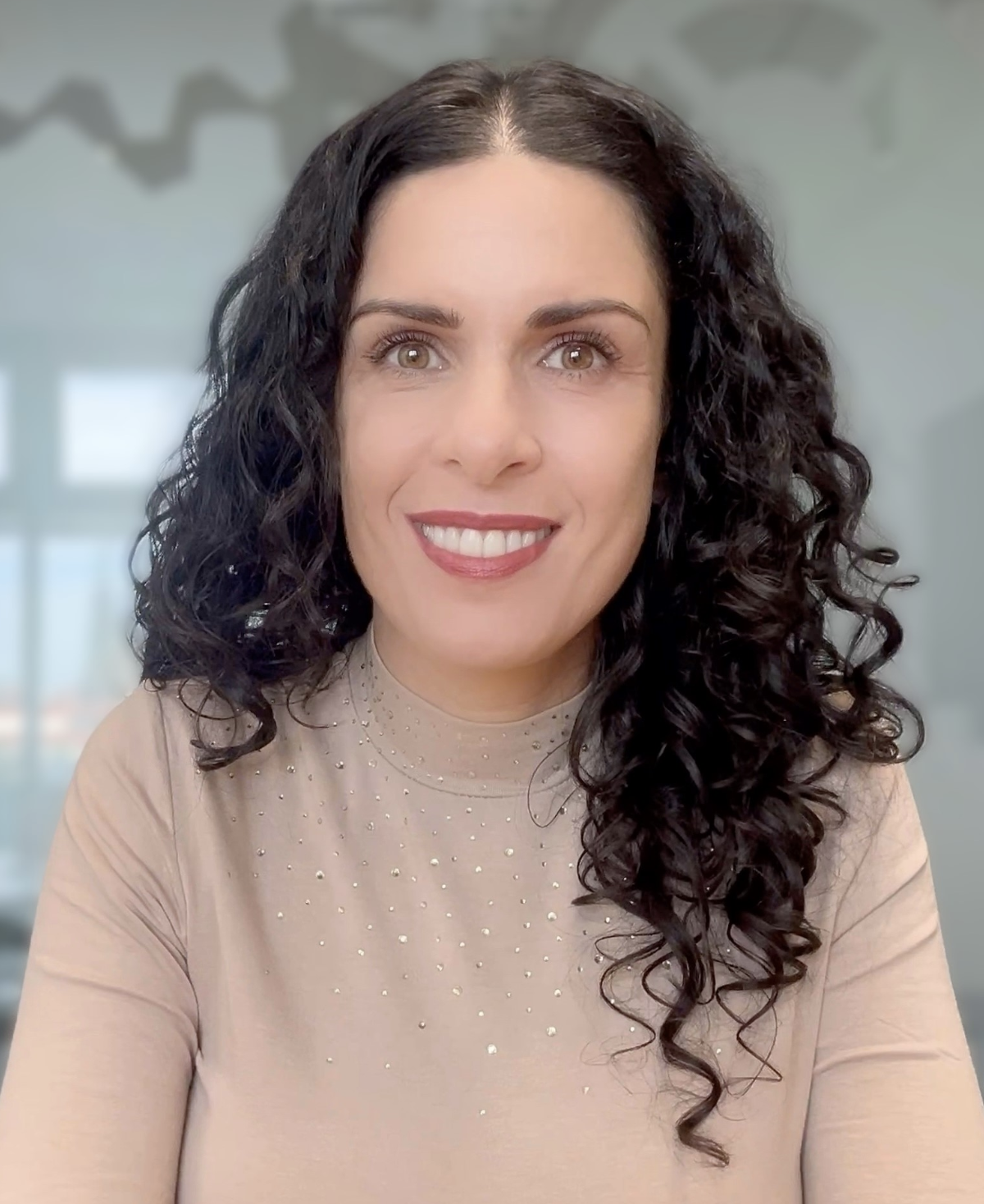
Marina Pantcheva
I am a linguist and polyglot with a rich experience in academic pursuits (research, teaching and science popularization) as well as management, leadership and innovation. I hold a PhD degree in Theoretical Linguistics. My academic work centered around the exploration of the elementary particles of language within the innovative framework of Nanosyntax. In 2014, I transitioned to the fast-paced world of Localization. Over the course of several years, I led a team developing processes and solutions for crowd-based localization, covering technology, BI, linguistic quality, Community management and more. Currently, I am heading the Linguistic AI Services Center of Excellence at RWS, dedicating my efforts to the development and implementation of linguistic AI solutions. I am a fervent advocate for the use of clear language. I am equally passionate about knowledge sharing and am frequently involved in outreach initiatives, such as public presentations, blog contributions, podcasts and other events dedicated to the dissemination of knowledge. In my spare time, I paint, read, and engage in research inspired by the vast amount of data I encounter in my daily work.
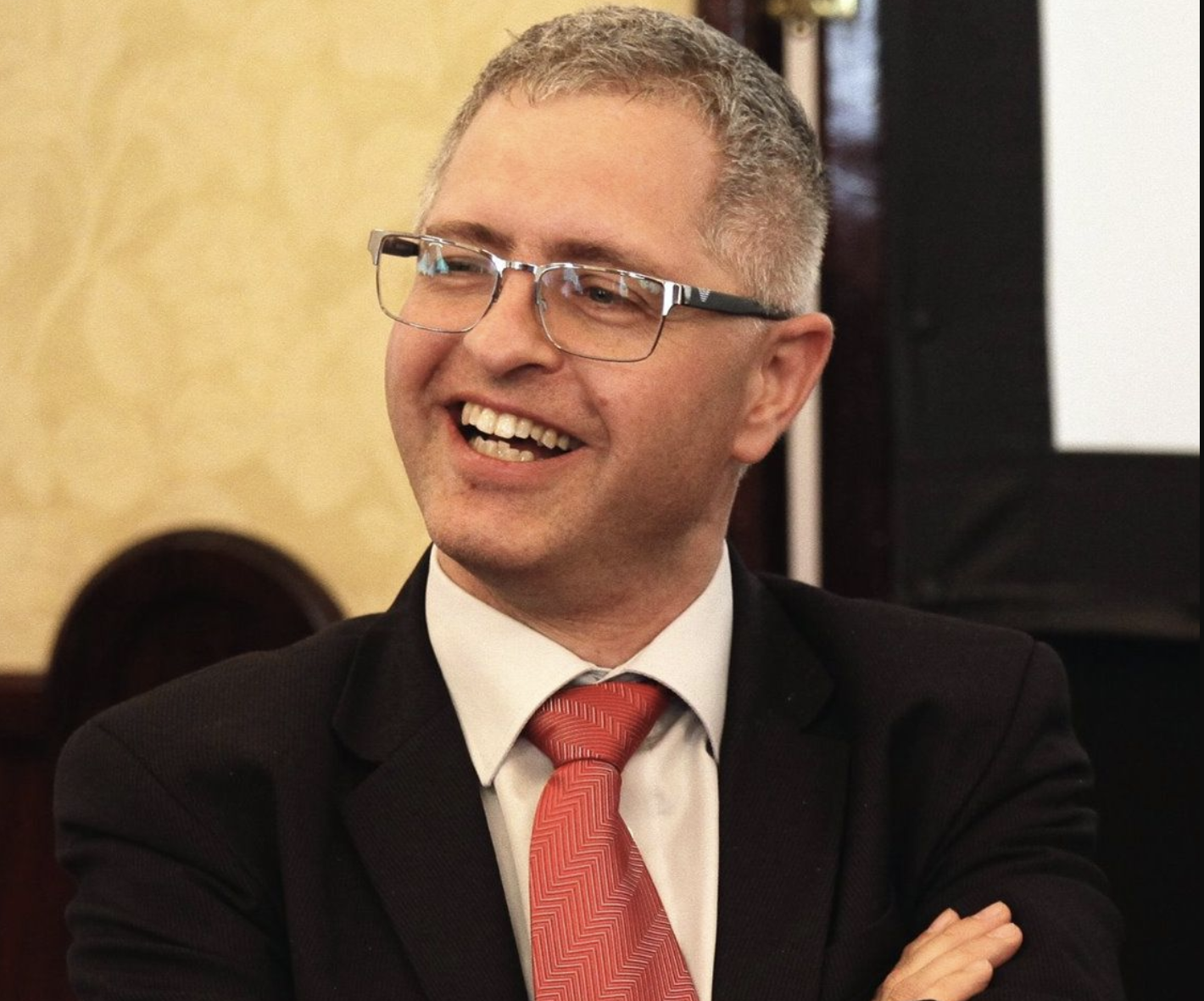
Balázs Kis
Balázs Kis is Chief Evangelist of memoQ. He is also one of the founders of the company. Balázs has decades of experince in IT, translation, and natural language processing. He has a degree in IT engineering and a PhD in applied linguistics. At the start of his career, he was a Microsoft systems engineer and trainer and one of the prominent Hungarian IT authors with over 20 titles published. He was also the head of research and development at MorphoLogic, a Hungarian company specializing in language technology research. He taught translation technology at the ELTE University of Budapest. He has massive experience in collaborative translation and project management. In the early years of memoQ, he was instrumental in product design - he is the author of the first design document of memoQ - and running the company. Later on, he became responsible for technical communication. Since memoQ became a shareholding company in 2016, he has been chairman of the board. From 2018, he was responsible for compliance matters at the company, until, in 2020, he was appointed one of the co-CEOs of the company. He recently moved on to the more outward position of the Chief Evangelist. Balázs is passionate about educating both the professionals and the general public on translation, localization, and the technologies related to them.