- Home
- Resource Center
- Articles & Videos
- Responsible Use of AI: The Key Role of Non-English Linguists
18 September 2023
| by Ushuaia Solutions
Responsible Use of AI: The Key Role of Non-English Linguists
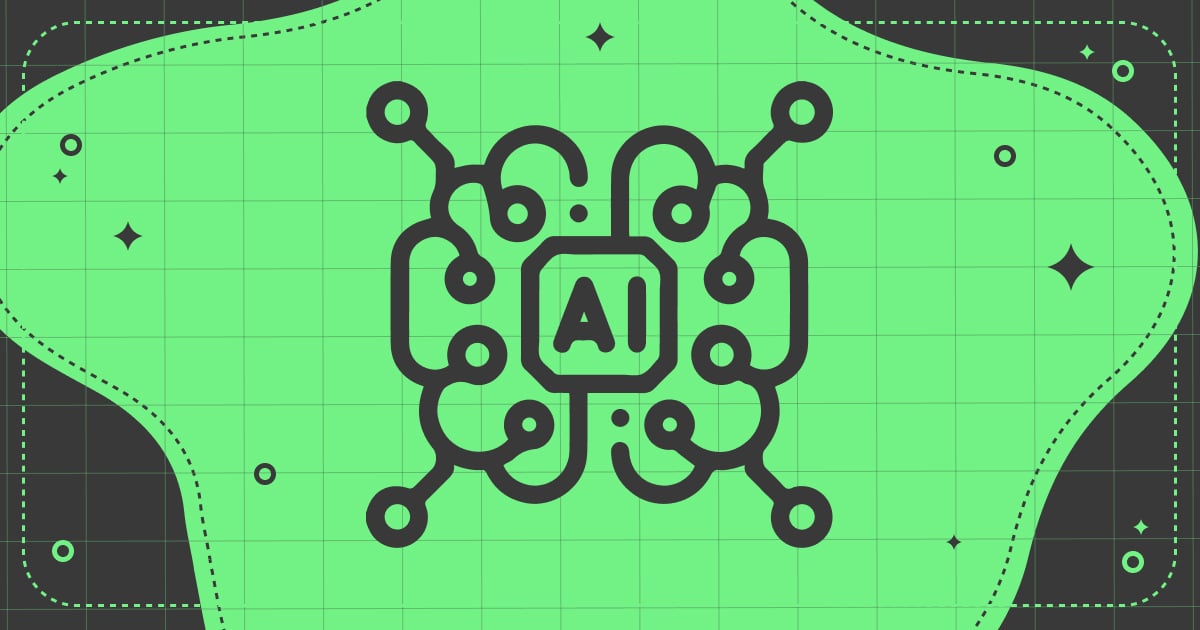
Sign up for our newsletter on globalization and localization matters.
I recently came across a meme: "Keep calm and stop explaining that AI will not replace translators". While the formula may be a little bit 2000, it was right on point.
Let’s try a different approach. I will encourage you to think of the obvious intersection between generative AI and existing language services not as a one-way effect or an end, but as a virtuous circle, a means to enable a more responsible and fair use of generative AI technologies.
Think of AI not as a technology that can be used to replace, enhance or forever poison the translation process, but as a new mechanism (for lack of a better word) that will require the ongoing services of language experts to fulfill its ethical use.
Responsible Use of AI and Equal Opportunity
In the midst of facts, warnings, opinions and forecasts, both for and against, the big question about the responsible use of AI remains a very pressing topic. As we speak, legislation is being passed around the world about how to regulate and implement new policies on safety, privacy, fairness and transparency in the use of generative technologies.
The benefits of the technology are huge, and so are the risks of potential misuse. Companies developing generative AI Application Programming Interfaces (APIs) are investing a lot of money in getting it right. In English, and in other languages. "What an exciting time to be in the language industry", everyone keeps saying.
Underrepresentation of Non-English Languages in AI:
A New Challenge for Society, an Opportunity for Linguists
It may not come as a big surprise to non-English speakers, but the fact is that a very high percentage of the efforts being made on responsible use of AI are for English as Language 1. On-going processes such as rating, categorizing, filtering, and moderating content, both for prompts and responses, start with Language 1. Therefore, ideally in the very near future, much of this effort will be -or is being- localized into other languages in the hope of being able to apply the same categories to other languages and cultures.
Now wait a second. Is this a new content type with very specific requirements and use cases that will be used to set the tone and train future similar cases? Does this content type need to be translated, adapted and re-categorized? Can we tell the difference between original content and translated content? Does it even matter? I am tempted to dwell on the philosophical implications, but this may not be the place, (or is it?).
It turns out that as language specialists –or better yet, as experts in multilingualism– we are not only in a unique position to ensure the correct use of grammar, syntax and terminology, but also set to be key players in the implementation of safety guidelines and training Large Language Models (LLMs) to identify content that is biased, violent, toxic or harmful to non-English languages, which –no surprise here– are still underrepresented in Generative AI. For this audience, it goes without saying that there will be a lot of adaptation and localization involved in the process.
In short: The more we learn about the use cases, the biases, and how LLMs work, the better we can serve these new emerging services.
Where Can LLMs Go Wrong and How Can Linguists Help?
There are many examples of errors and biases in AI language models. The role of linguists and experts is crucial to correct mistakes and ensure a responsible use of the technology. Let’s analyze some of the most common problems with AI.
Edge Cases: Edge cases refer to unusual, rare, or exceptional situations that are not well represented in the training data. These cases can lead to limitations in the performance of generative AI APIs, such as model overconfidence, misinterpretation of context, or inappropriate outputs. What can we do? Flag and categorize responses. Rewrite responses to fit the purpose.
Model Hallucinations, grounding, and factuality: Produced content may lack grounding and factuality in real-world knowledge, physical properties, or accurate understanding. This limitation can lead to model hallucinations, which refer to instances where the technology may generate outputs that sound plausible, but are factually incorrect, irrelevant, inappropriate, or nonsensical. Understanding how the technology works and being able to flag these cases is essential. While one could argue that a native speaker can do this, trained linguists are in a unique position to perform this and other similar tasks.
Data quality and tuning: The quality, accuracy, and bias of the prompts and/or data inputted into the apps can have a significant impact on their performance. If users enter inaccurate or incorrect data or prompts, the generative output may show suboptimal performance or false model outputs. What can we do? Create model prompts to increase the range of good responses.
Bias amplification: Language models can inadvertently amplify existing biases in their training data, resulting in outputs that may further reinforce societal prejudices and unequal treatment of certain groups. What can we do? Flag and rewrite.
Language quality: The majority of benchmarks (including all of fairness evaluations) are in the English language. What can we do? Review the outputs for grammar, terminology, syntax, and fluency in the target languages or in multilingual models. Language models may provide inconsistent service quality to different users. For example, text generation might not be as effective for some dialects or language varieties due to underrepresentation in the training data. Performance may be worse for non-English languages or English language varieties with less representation. Don’t you sense the opportunity here?
Fairness benchmarks and subgroups: Fairness efforts focus on biases along the axes of gender, race, ethnicity and religion, but perform the analysis only on the English language data and model outputs. Other minorities and languages are probably underrepresented and will need further input. What can we do? Flag and introduce new content. Translate content to increase the representation of minority groups in LLMs. Review content for inclusivity and accessibility.
Limited domain expertise: APIs may lack the depth of knowledge required to provide accurate and detailed responses on highly specialized or technical topics, resulting in superficial or incorrect information. For specialized, complex use cases, APIs should be tuned on domain-specific data, and there must be meaningful human supervision in contexts with the potential to materially impact individual rights. What we can do: provide SMEs with underrepresented (I am tempted to say target) languages.
Length and structure of inputs and outputs: APIs can handle a wide variety of content types and structures, but they have length and structure limitations that may lead to poor model performance. What can language experts do? Review and validate content for correct structure based on content type, including character limitations and featured information (i.e. posts, tweets, ads, articles, banners, abstracts) in non-English languages.
A Bright Future for Linguists
When taking a closer look, it seems that the human use of generative technologies is opening the door to new challenges in terms of effective and responsible communication, discourse legitimation, protection of vulnerable groups and prevention of violent speech. The massive implementation of generative technologies makes it essential to cope with these challenges in many languages, across many countries, using both a global and very local approach. Linguists of the world, do not fear! There is still a lot of (new) work to be done. You have a key role to play, make it count!
Do you want to contribute with an article, a blog post or a webinar?
We’re always on the lookout for informative, useful and well-researched content relative to our industry.

Agustina di Pratula
Agustina, CEO at Ushuaia Solutions, is a seasoned professional in the localization industry. With a background in languages, she entered the localization industry more than 20 years ago. Immediately interested in the vast world of language technologies and global organizations and after a brief period as a linguist, she quickly moved towards project management and leading the Operations team. She has deep connections with industry colleagues both locally and internationally. Agustina's personal interests extend to the arts, especially the visual arts, including the creation and exhibition of her own artistic endeavors.